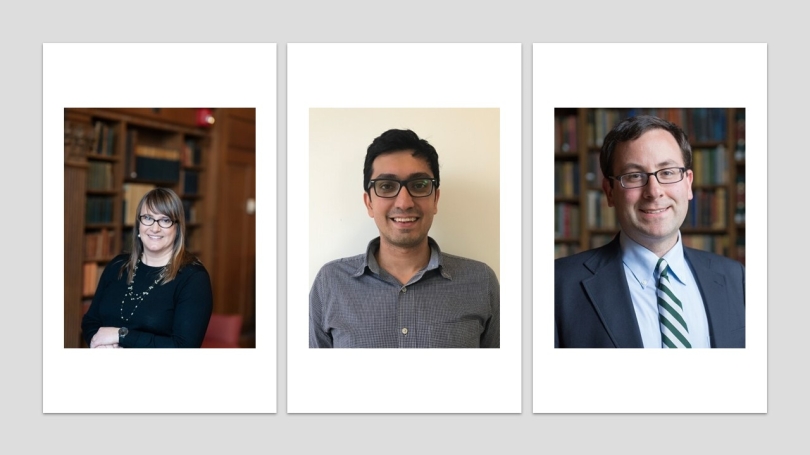
- Undergraduate
- Research
- About the Program
- News & Events
- People
Back to Top Nav
Back to Top Nav
Back to Top Nav
Back to Top Nav
Professors Jacqueline Wernimont (WGSS), Soroush Vosoughi (Computer Science), and Jed Dobson (ENG) recently took part in a panel on ChatGPT and language learning. You can read about the panel here.
We asked each professor to comment on how generative artificial intelligence and ChatGPT in particular might affect the Program of in Quantitative Social Science (QSS) and the classes taught in it, and here is what they had to say.
According to Professor Wernimont, "Tools like ChatGPT have the ability to help us cut down the time we spend doing some formulaic and rote tasks, but only once we've learned how to do those tasks ourselves. I think the qualifier - once we've learned how to do those tasks ourselves- is really critical. The effectiveness of a tool depends on the skills of the person wielding it and AI tools sometimes create the illusion that we don't need to do the hard work of understanding how they work before we use them."
Professor Vosoughi wrote that, "I can see QSS facing the problems of other disciplines with respect to ChatGPT-supported plagiarism (e.g., students using ChatGPT to generate code). However, ChatGPT can also be a valuable asset to the QSS program. Given the interdisciplinary and unique nature of QSS, ChatGPT can be of great assistance for students to explore connections between fields, identify relevant literature, and answer questions about different applicable quantitative methods. I can see ChatGPT being integrated into the QSS course more seamlessly than many other programs."
Professor Dobson, who teaches QSS 30.16, a course on text analytics, write that, "Large language models and applications built on them like ChatGPT present both opportunities and challenges for QSS. These new models offer state-of-the-art performance on many natural language processing tasks and can be used for modeling, classifying, and summarizing many of the text sources of interest to students and researchers in QSS. There is also much exciting work to be done to understand how these tools model language and how they make decisions. Large language models have also become quite good at generating text to fit certain genres and constraints, especially when used in the production of code. Like the problems facing writing instructors, faculty are returning to the question of what they want from students in courses organized around producing code. We may be increasingly prioritizing metacognition and reflection on the transformations and operations embedded in the code in terms of the theories motiving the present intellectual project, the choices made by the researcher, and the histories informing these methods."